Two groups of final year Mechanical Engineering students at the University of Bristol spent the first half of 2021 working on Digital Twin-based projects. The students have used many of the tools, methods, and expertise within the DETI consortia to design, develop and implement from first principles the foundations of Digital Twins for a product (Brompton Bike) and a production system (future bicycle factory).
The product-focussed digital twin combines physics-based models of aerodynamic, structural and human performance with ride quality to provide a holistic digital emulation environment supporting the redesign and personalisation of current products as well as exploration of future models. To create the Digital Twin the models are integrated by journey type and route details; rider anthropometrics and riding style to allow the simulation of an entire journey; or a set of journeys and annual commute. Alongside multi-physics simulation, principles of model-based systems engineering (MBSE) were applied through collaboration with colleagues at CFMS to create the foundations for the Digital Twin.
Screenshots of the models and the architecture and user interface of the digital twin are depicted in figures 1 and 2.
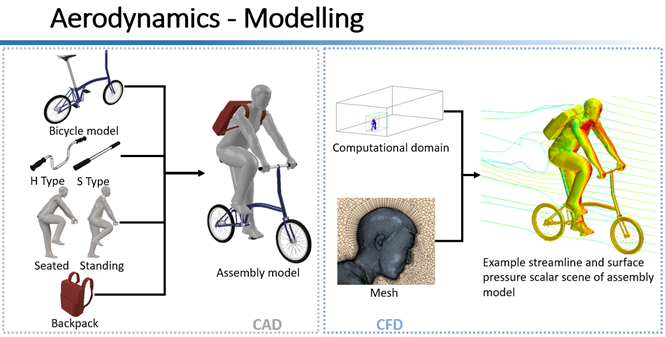
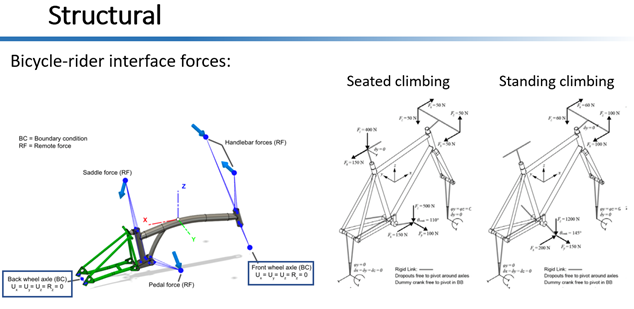
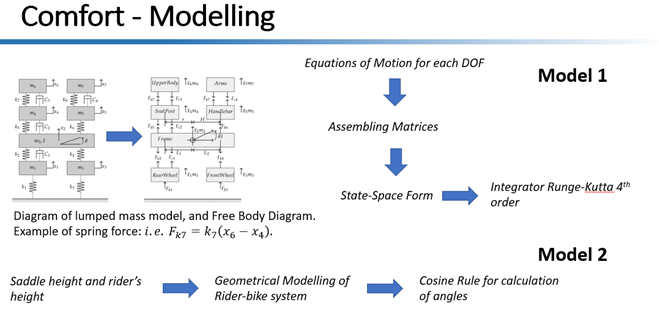
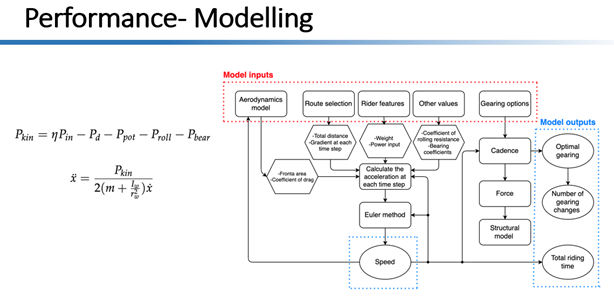
Figure 1 – Multi-physics models of the components of a digital twin
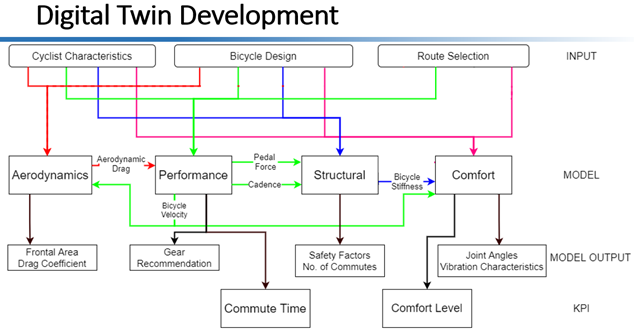
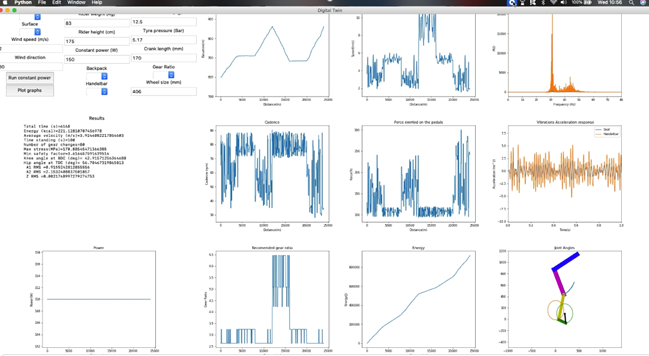
Figure 2 – Digital twin architecture (threads) and interface
Due to the pandemic, real-time monitoring of the bike was not possible, so historical data was used. The real-time data links will be investigated by next year’s student who will also be looking at the inclusion of an electric drive (motor and battery pack).
The production-focussed digital twin aimed to create a digital twin for a virtual carbon-fibre racing bicycle factory. The improvement provided by the digital twin to individual purposes within the factory was explored. Through this, insights into a general methodology for designing digital twins were gained.
Four purposes were chosen to be incorporated within the digital twin: Defect Detection, Predictive Maintenance, Inventory Management, and Internal Disruptions Management. A preliminary study was conducted to determine the necessary data and data acquisition methods for each purpose.
The Defect Detection purpose sets out a method of using relationships between defects and process conditions to improve defect detection and reduce defect rate. Due to the complex and highly variable nature of carbon fibre reinforced polymer manufacturing, these relationships do not yet exist for many defects and so calculations are completed to determine necessary sample sizes for forming such relationships during factory operation. Predictive maintenance was explored to maximise the usable lifetime of machines in the factory and minimise downtime. Two statistical models were used to predict the remaining useful life of machines in the factory. These models were tested against time-based-maintenance using 648 failure profiles. The digital twin used these results to select appropriate models given a machines historical performance. Within the inventory management purpose, three machine learning models were developed and trained using historical sales data to predict future demand. The best performing machine learning algorithm, a Random Forest model, had 10.9%, and 5.8% lower mean absolute and root- mean-square errors on the test data relative to the best traditional forecasting method. Internal Disruptions Management used a heuristic algorithm to calculate improved configurations of workers following a disruption. This provided average improvements to factory throughput of between 3.81% and 18.34% for disruptions between 120 and 480 minutes respectively.
The overall approach taken in the project and results are shown in Figures 3 to 6.
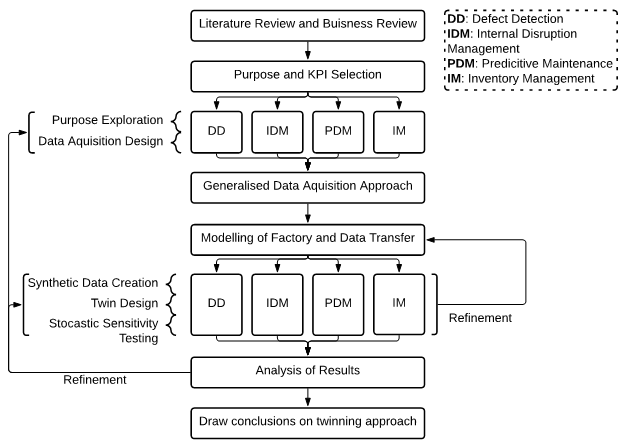
Figure 3 – Factory digital twining approach
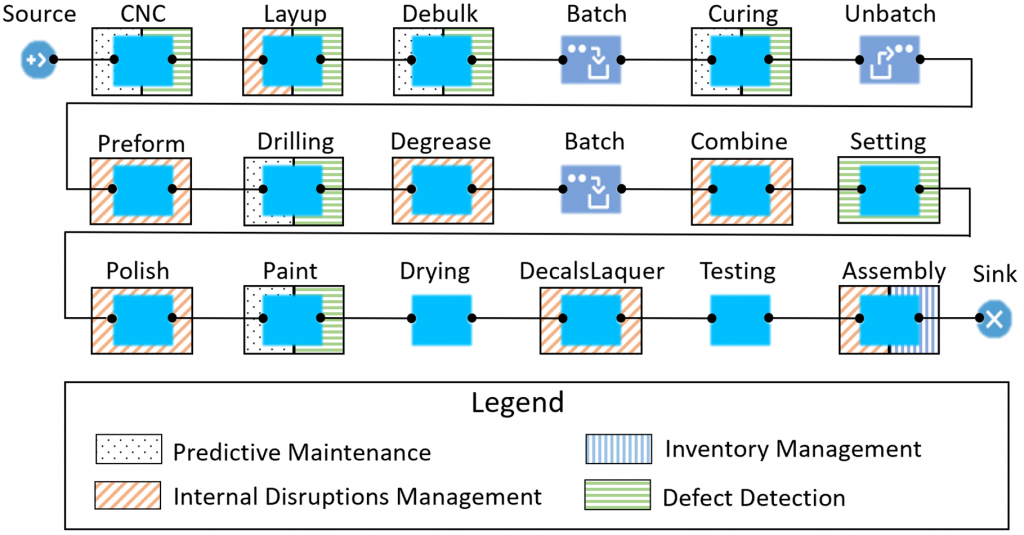
Figure 4 – Factory model with different purposes indicated
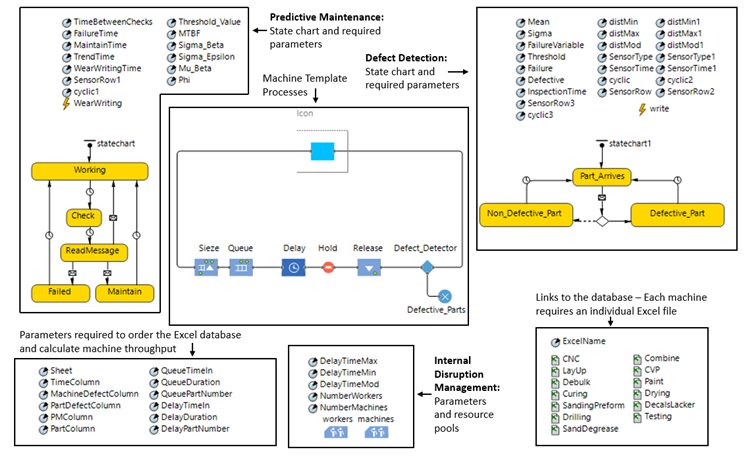
Figure 5 – Agent-Based template for each process stage
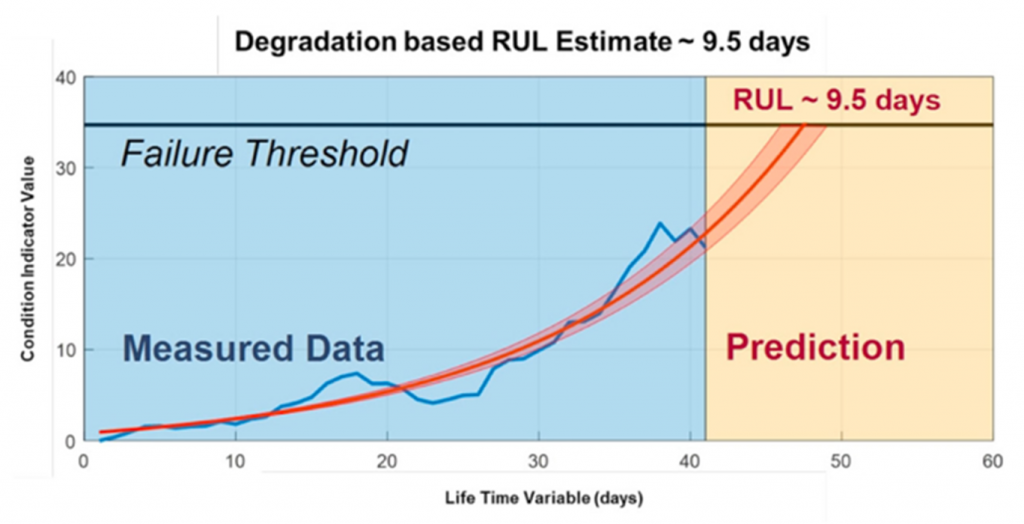
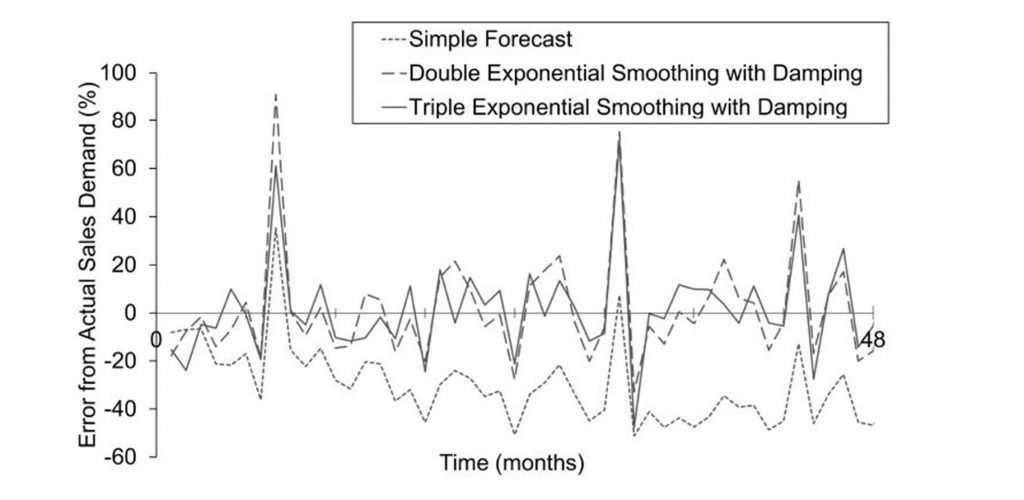
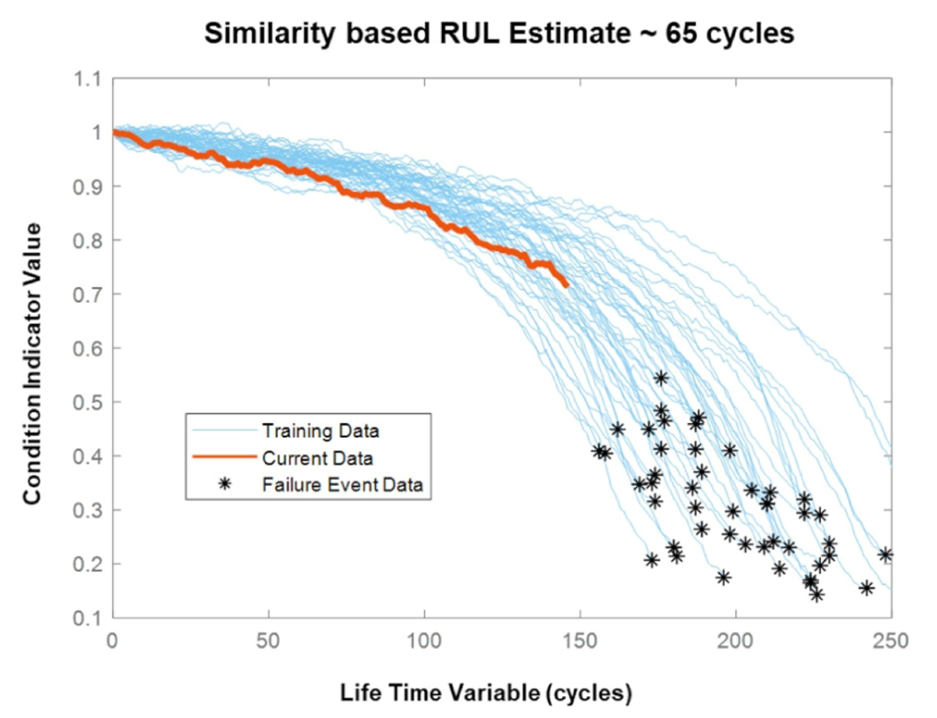
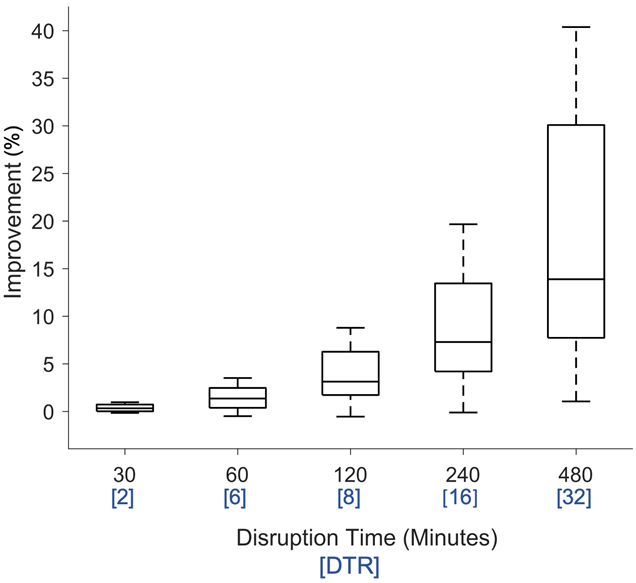
Figure 6 – Some of the twinning-based outcomes
The University of Bristol has a number of Training factory industry 4.0 kits coupled with Industrial Siemens PLCs which can also be linked to virtual factory models using FactoryIO. We are looking for industrial test cases to model using this equipment to create impactful demonstrations for digital-physical cyber-physical systems; please get in touch if you are interested in a low-risk approach to explore the possibilities of what Industry 4.0 may be able to do for you.
The projects were undertaken as part of the partnership between the University of Bristol and CMFS involving Dr James Gopsill. Ian Risk (CTO @ CFMS) commented on the importance of training engineering students in these state-of-the-art tools and methods, and the relevance and timeliness of the research projects for the work being undertaken within the DETI programme.
For further information of enquiries please contact:
Professors Ben Hicks or Aydin Nassehi (University of Bristol)
Dr James Gopsill (CFMS)