This project tests the hypothesis that Cyber-physical production systems can be developed much faster using “non-identical digital twins”. This is achieved by creating a lightweight, low fidelity, digital twin to a physical system and co-evolving this twin, together with adjusting, the parameters in the real system. The co-evolution is enabled by learning from both the digital and the physical twins and shaped further by input from domain experts. Techniques such as machine learning and sensor data fusion are used together with automated rigs that emulate manufacturing processes to inform the research and allow the mechanical properties of the process to be assessed in both the physical and cyber environments.
What is it all about?
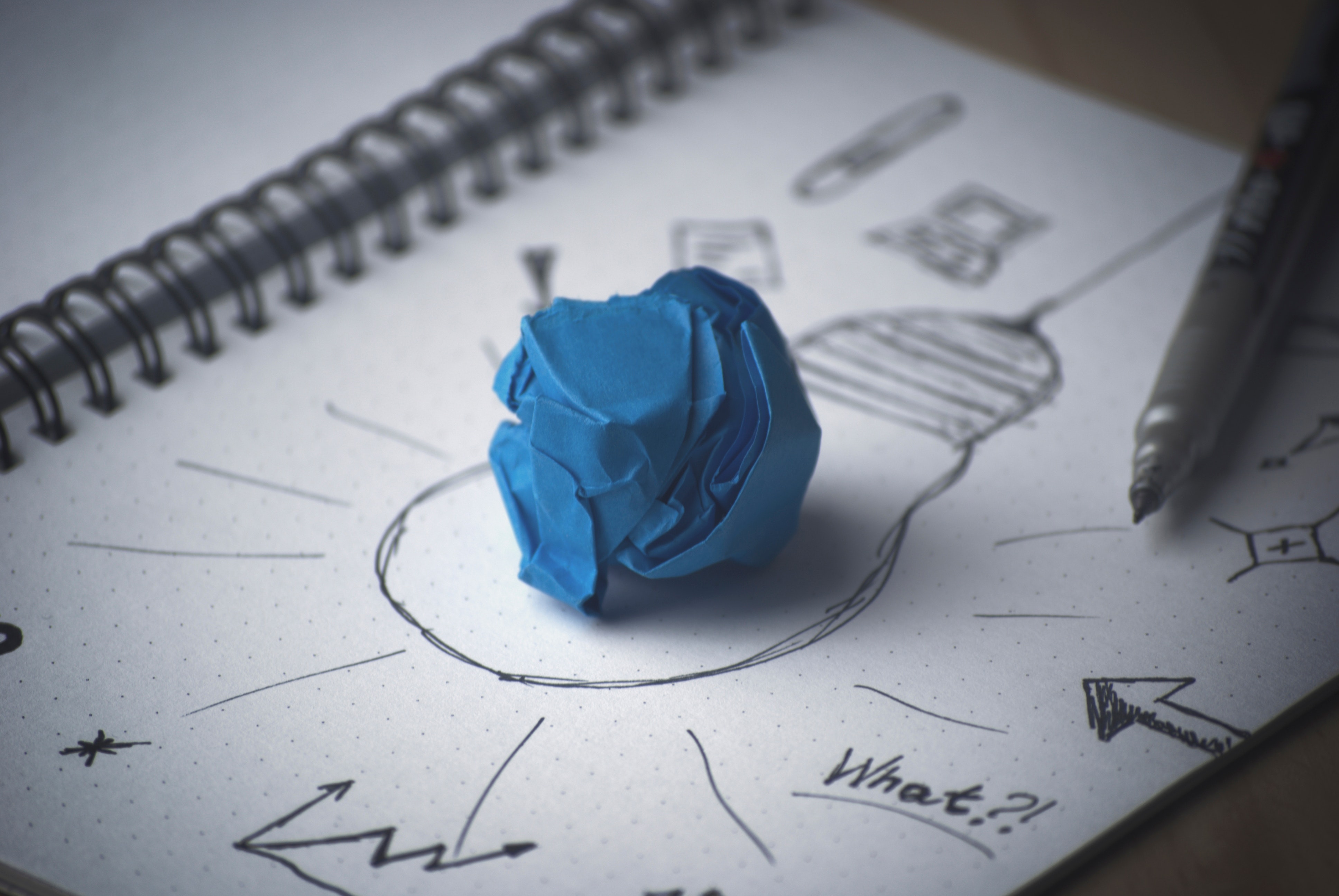